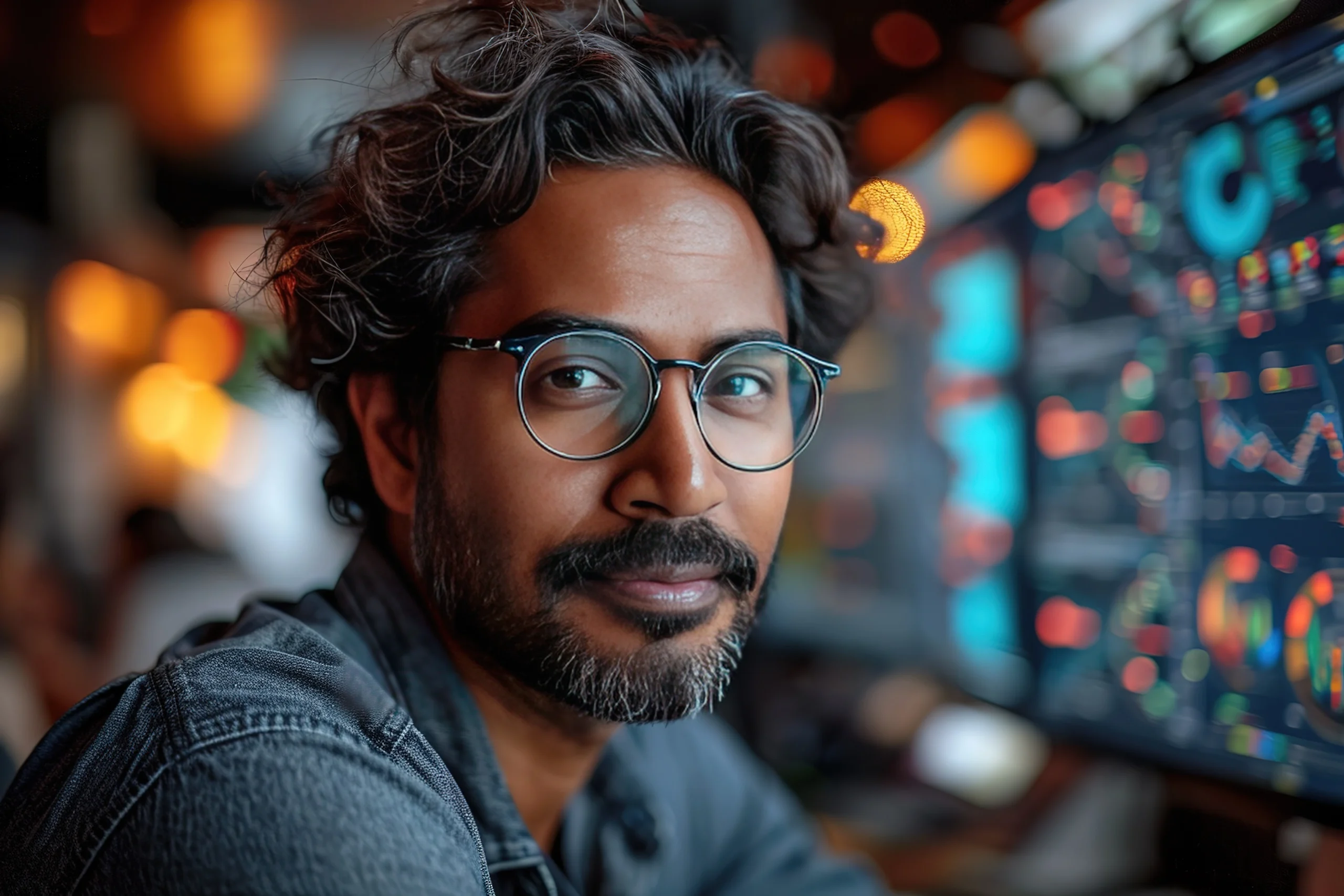
In this article, we consider the similarities and differences between supervised vs unsupervised learning. In supervised learning, the algorithm is given labelled data, meaning it is provided with input-output pairs. The goal is to build a predictive model from this data, teaching the algorithm to recognise patterns and make accurate predictions for new, previously unseen data. On the other hand, in unsupervised learning, the algorithm is given unlabelled data, where it is tasked with discovering patterns and structures in the data itself without any guidance.
Emergence of supervised vs unsupervised learning
Supervised learning has its roots in the 1950s, when researchers began developing algorithms capable of recognising patterns in data and making predictions based on these patterns. The emergence of digital computers at the time accelerated the development of these algorithms. Unsupervised learning, on the other hand, received more attention in the late 1980s and 1990s, when researchers started experimenting with neural networks and clustering techniques to analyse and understand unlabelled data.
TriFact365 uses both supervised and unsupervised learning to help its customers automate and optimise their invoice processing processes.
Laws and regulations
It is important to understand the differences between supervised and unsupervised learning. The genesis and relevant laws and regulations are also relevant. This will help you gain a better understanding of how these techniques are applied and used in various application areas. The use of supervised and unsupervised learning is subject to different laws and regulations. Especially in the areas of privacy and ethics. Organisations deploying these techniques must ensure that they comply with applicable laws and regulations. Consider laws such as the General Data Protection Regulation (GDPR) in Europe. Other laws and regulations apply in other parts of the world. These regulations are designed to protect individuals’ privacy. They also ensure that their data is used responsibly when training machine learning models.
Why you want to work with this
In a world where data drives decision-making, supervised and unsupervised learning play a crucial role. Especially if you want to gain accurate insights from your data to make strategic decisions. By using supervised learning, patterns in your data can be identified. Predictive models can then be developed. This allows you to optimise business processes and gain competitive advantage.
How to put this to good use
Successfully deploying supervised and unsupervised learning requires a good understanding of your business objectives and available data. Start by identifying specific problems or challenges. Determine which technique is best suited to address them. Make sure you have sufficient qualitative data to train your models. Keep iterating and optimising to improve the accuracy and relevance of your results.
Shortcomings and hazards
While supervised and unsupervised learning are powerful tools, they also have some shortcomings and dangers. With supervised learning, for example, it is essential to have enough labelled data, which can be difficult to obtain in some cases. There is also the risk of overfitting, where the model is overfitted with training data and therefore makes poor predictions for new data. With unsupervised learning, there is the risk of discovering unintended patterns or biases in the data. This can lead to wrong conclusions or decisions.
How TriFact365 uses supervised vs unsupervised learning
TriFact365 uses both supervised and unsupervised learning to help its customers automate and optimise their invoice processing processes. With supervised learning, accurate models can be developed to automatically recognise, classify and validate invoices. This can reduce processing time and minimise errors. In addition, TriFact365 uses unsupervised learning to gain insights from large amounts of invoice data. This allows customers to better understand where their spending is going and how to further optimise their financial processes.